Modeling guide for routing problems¶
List variables are a very powerful modeling feature for various problems where collections of items have to be ordered in a optimized fashion. For instance, scheduling problems, production planning problems, crew scheduling problems or even assignment problems can be efficiently modeled and solved with list variables.
State-of-the art results can also be obtained for routing problems modeled with list variables. Starting with the traveling salesman problem, this page covers most routing optimization variants and explains how it can easily be modeled with LocalSolver.
Note
The most common routing problems, namely TSP, CVRP and CVRPTW are available in our example tour as complete models with sample data and ready-to-use code in LSP, C++, Java, .NET and Python. This guide refers the reader to this pages and also explore other features like pickup-and-delivery constraints, waiting time minimization, prize-collecting variants, and so on.
The Traveling Salesman problem (TSP)¶
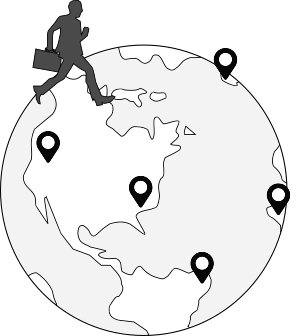
The simplest routing problem involves only vehicle, meant to visit a given set of cities with the smallest possible tour.
This problem is easily modeled with a single list variable constrained to contain all cities. Below is the 3-line LSP model for the travelling salesman problem. Despite its simplicity, this model yields high-quality solutions very quickly. See our benchmark page for a performance comparison with MIP solvers. Complete models for LSP, C++, Java, .NET and Python are available in our TSP example.
// A list variable: cities[i] is the index of the ith city in the tour
cities <- list(nbCities);
// All cities must be visited
constraint count(cities) == nbCities;
// Minimize the total distance
minimize sum(1..nbCities-1, i => distanceWeight[cities[i-1]][cities[i]])
+ distanceWeight[cities[nbCities-1]][cities[0]];
The Prize-Collecting Traveling Salesman problem (PCTSP)¶
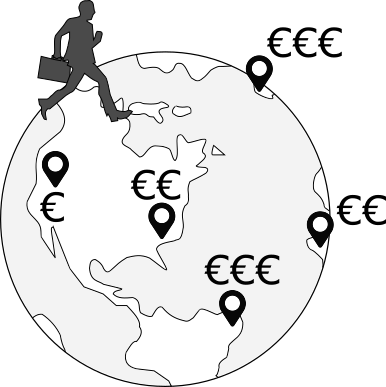
Sometimes visiting all the cities is not mandatory. In the prize-collecting traveling salesman problem, a revenue is attached to each city and the objective is to maximize the net revenue defined as the total collected revenue minus the total traveled distance (counting a cost of 1 per unit of distance).
This variant requires only a slight modification of the TSP model, as illustrated below.
// A list variable: cities[i] is the index of the ith city in the tour
cities <- list(nbCities);
revenue <- sum(cities, i => prize[i]);
distance <- count(cities) <= 1 ? 0 : sum(1..count(cities)-1, i => distanceWeight[cities[i-1]][cities[i]])
+ distanceWeight[cities[count(cities)-1]][cities[0]];
maximize revenue - distance;
You can observe that the contraint on the size of the list has been removed. Consequently the distance expression is modified to take into account the now possible case of empty or singleton lists. The revenue collected by the tour is measured with a simple sum. Note that the range on which the sum is applied can be defined as an interval (as in the distance case, to enumerate all positions in the list) or directly as a list (to enumerate values contained in the list).
The Capacitated Vehicle Routing Problem (CVRP)¶
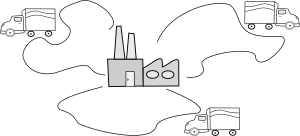
The objective is to assign a sequence of customers to each truck of the fleet minimizing the total distance travelled such that all customers are served and the total demand served by each truck does not exceed its capacity.
As explained in our example tour this problem is modeled with one list variable per truck of the fleet, and the coverage of all customers is unsured with a partition constraint:
// All customers must be visited by the trucks
constraint partition[k in 1..nbTrucks](tour[k]);
And the capacity contraints is modeled with a sum over all items assigned to a truck:
sequence <- tour[k];
c <- count(sequence);
routeQuantity <- sum(0..c-1, i => demands[sequence[i]]);
constraint routeQuantity <= truckCapacity;
Many test cases of this capacitated vehicle routing problem are available in the literature. Our benchmark page shows how quickly LocalSolver finds high-quality solutions to these problems.
The Prize-Collecting Capacitated Vehicle Routing Problem (PCCVRP)¶
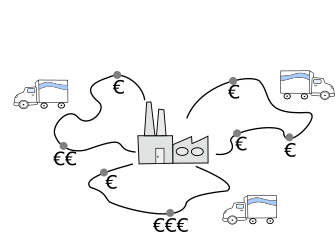
In this context, the lists will not form a partition of the set of all customers, but it is still forbidden to have two trucks or more visiting the same customer. Therefore the partition contraints becomes a disjoint constraint:
// At most one visiting truck per customer
constraint disjoint[k in 1..nbTrucks](customersSequences[k]);
The remaining of the model is unchanged. Only the objective function now
measures the total collected revenue, defined on each tour as
sum(tour[k], i => prize[i])
.
The Capacitated Vehicle Routing Problem with Time-Windows (CVRPTW)¶
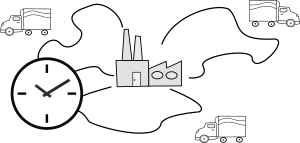
In many cases (for example in the standard CVRPTW), it can be modeled without introducing delivery times as decision variables. Indeed, a key modeling principle is to avoid introducing a decision when an expression can in fact be inferred from other expressions. It is the case for the CVRPTW, where the delivery time for a customer can be defined as the maximum of:
- the earliest allowed delivery time for this customer
- the travel time from the previous customer added to the departure time from the previous customer
Such a recursive definition is introduced with a function (i, prev) => ...
.
It allows defining the ith element of an array in function of i and of the
(i-1)th element of the array. See our
documentation on this topic for details:
endTime[k] <- array(0..c-1, (i, prev) => max(earliestStart[sequence[i]],
i == 0
? distanceWarehouse[sequence[0]]
: prev + distanceMatrix[sequence[i-1]][sequence[i]])
+ serviceTime[sequence[i]]);
The lateness at each visit is computed from the difference between the ending time of this visit and the latest allowed end for this customer. For the arrival at the depot, we compare the arrival time to the maximum horizon defined for the problem.
Our example tour includes a detailed model for this problem with sample data and ready-to-use code in LSP, C++, Java, .NET and Python.
CVRP with preassignments¶
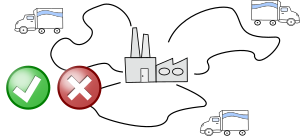
Such constraints can easily be defined on list variables thanks to the
contains
operator:
// with mandatory and forbidden defined as maps of pairs truck/visit
for[req in mandatory] constraint contains(tour[req.truck], req.visit);
for[req in forbidden] constraint !contains(tour[req.truck], req.visit);
The Pickup and Delivery Problem (PDVRP)¶
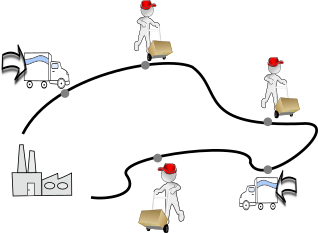
This situation induces three constraints:
- Each pickup/delivery pair must be assigned to the same tour
- Within this tour, the pickup cannot be visited after the delivery (we cannot deliver and item that has not been picked up yet)
- the weight of the truck will vary along the tour (increasing upon pickups and decreasing upon deliveries) and the capacity constraint must be satisfied at any time during the tour.
Considering each list in the LocalSolver model, the first requirement for a
pickup/delivery pair (a,b) means that a and b either both belong to the list or
none of them belong to the list. Literally it means that
contains(sequence[k], a) == contains(sequence[k], b)
.
As for the ordering of the pair within the list, it is related to the position
of a and b within the list, which can be obtained with the indexOf
operator:
for[k in 1..nbTrucks] {
constraint contains(sequence[k], a) == contains(sequence[k], b);
constraint indexOf(sequence[k], a) <= indexOf(sequence[k], b);
}
Note that indexOf takes values -1 when searching for an item that is not contained in the list. Consequently writing a strict inequality on the second constraint would be an error because it would not allow both items to be outside of the list.
As mentioned above, the last specificity is that the capacity constraint must now be checked after each visit. To do this, we need to define the weight after a visit as the weight before the visit plus the weight of the current item (positive if loaded, negative if delivered):
for[k in 1..nbTrucks] {
weight[k] <- array(0..c-1, (i, prev) => prev + demands[sequence[i]]);
constraint and(0..c-1, i => weight[k][i] <= truckCapacity);
}
CVRPTW with minimization of waiting time¶
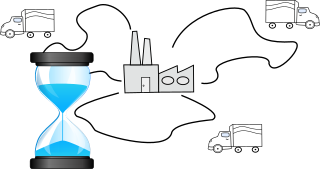
However, this strategy can lead to unnecessary waiting time for drivers. Hence and additional requirement is sometimes to minimize the total waiting time, or (equivalently) to minimize the total duration of tours.
A simple approach can be to introduce a targetEndTime[j]
integer decision
variable for each visit. In this case it will be important to consider that the
ending of a visit is not directly targetEndTime[j]
but instead the maximum
between targetEndTime[j]
and the earliest arrival at j from the previous
visit.
Alternatively, we will show below that introducing these decisions can be
avoided, thanks to a careful computation of the minimum waiting time for a tour.
To achieve this, we compute the earliest scheduling of the tour as in the
classical CVRPTW and we maintain alongside the maximum shifting of the starting
time of the tour that is still possible without visiting a customer too late.
This margin
array is recursively computed based on the following definitions:
- We define arrival as the earliest arrival time on site (endTime of previous visit plus travelTime)
- We define the earliness as the time from arrival to the start of the time-window (0 if arrivalTime is within time-window)
- We define the slack as the difference between endTime and the end of the time-window
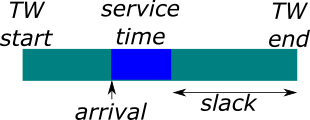
Regular arrival
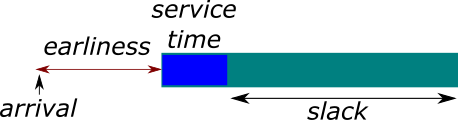
Early arrival
The rationale of the model is the following:
- If earliness is zero then we just observe the slack on this time-window. If smaller than the current margin, then we register this slack as the maximum possible shifting of the start of the tour:
margin <- min(margin, slack)
.- If earliness is non-zero then we try to absorb this earliness by shifting the starting time of the shift. If the current margin is larger than this earliness, then the residual margin is now
min(margin-earliness, slack)
. Otherwise we have not enough margin to avoid a waiting time ofearliness-margin
on this site. From this point, any earliness will be unavoidable waiting time.
The LSP code below implements this strategy. In this model, negative values of
margin[i]
code for the waiting time before visit i:
for[k in 0..nbTrucks-1] {
local sequence <- tour[k];
local c <- count(sequence);
// A truck is used if it visits at least one customer
truckUsed[k] <- c > 0;
endTime[k] <- array(0..c-1, (i, prev) => max(timeWindowsLB[sequence[i]],
i == 0
? travelTimeMatrixFromDepot[k][sequence[0]]
: prev + travelTimeMatrix[sequence[i-1]][sequence[i]])
+ handlingTime[sequence[i]]);
arrivalTime[k] <- array(0..c-1, i =>
i == 0
? endTime[k][i]-handlingTime[sequence[i]]
: endTime[k][i-1] + travelTimeMatrix[sequence[i-1]][sequence[i]]);
// If margin[k][i] > 0, it represents the remaining margin after visit i
// otherwise, -margin[k][i] is the waiting time for visit i
margin[k] <- array(0..c-1, (i, prev) =>
i == 0 ?
// We can always absorb the time on the first node
// (because we can delay the departure to not wait).
slack(k,0)
:
// Not the first node
prev > 0
// We still have some margin, we absorb earliness
? min(prev - earliness(k,i), slack(k,i))
// We don't have any margin, we wait if we arrive early
: -earliness(k,i));
// Total time that driver k waits on day d during his route
waitingTime[k] <- sum(0..c-1, i => max(-margin[k][i], 0));
}
function earliness(k, i) { return max(0,timeWindowsLB[tour[k][i]] - arrivalTime[k][i]);}
function slack(k, i) { return timeWindowsUB[tour[k][i]] - endTime[k][i];}
TSP with draft limits (TSPDL)¶
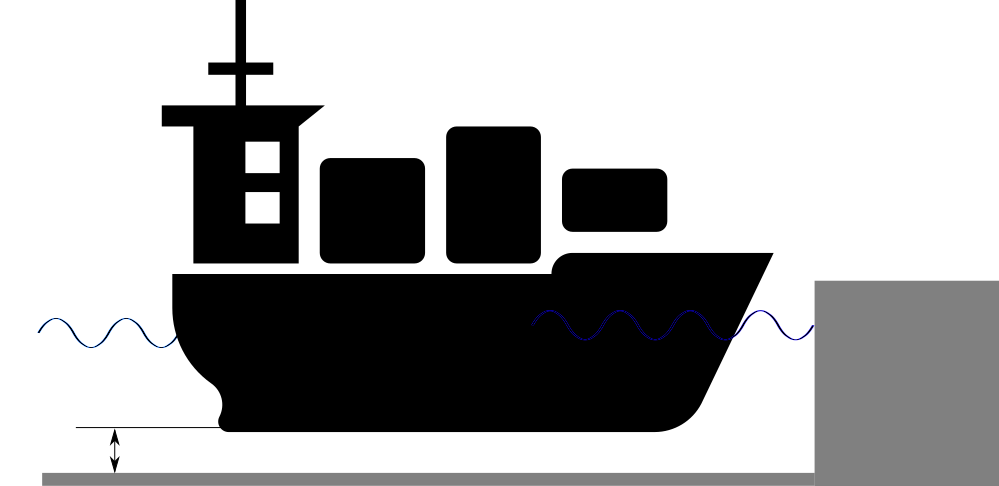
Such a limitation can occur in other contexts where the accessibility of a site depends on the weight of the vehicle. As in the pickup and delivery problem, it requires computing the weight of the vehicle along the tour:
weight <- array(0..c-1, (i, prev) => i == 0
? totalWeight
: prev - itemWeight[sequence[i-1]])
// Setting a contraint over all terms of an array is done with the 'and' operator:
constraint and(0..c-1, weight[i] <= weightLimit[sequence[i]])
// Altenatively (for instance if finding a solution respecting all draft limits
// take more than a few seconds), you can minimize the cumulated overweight :
overweight <- sum(0..c-1, max(0, weight[i] - weightLimit[sequence[i]]))
Other routing problems¶
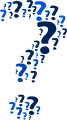